The Conservation Biology Institute’s recent work with the Deschutes Trails Coalition (DTC) and the Deschutes National Forest focuses on designing a Trails Assessment and Planning Tool for Deschutes County. We have developed a blueprint for the design, in collaboration with the U.S. Forest Service and the DTC. In this new phase of the project, funded by the U.S. Forest Service, CBI will partner with the DTC to build a prototype of the trails decision-support tool and sustainability model for Deschutes County. Then we will scale up and customize this prototype to meet the requirements of the U.S. Forest Service and its partners in the states of Oregon and Washington. The Trail Assessment and Planning Tool design includes creating a preliminary version of a sustainability framework that incorporates an interactive spatially-explicit model, addressing the physical, environmental, social, and economic aspects of sustainability. The model is powered by CBI’s Environmental Evaluation Modelling System (EEMS), allowing for its collaborative development with a diverse group of stakeholders, to create a transparent framework for local, regional, and national organizations to answer important questions relevant to trails planning and management.
Proxy Falls, Oregon
Michael Riffle / Flickr
CBI is working in collaboration with Oregon State University, the University of Minnesota, and the University of Colorado Boulder on a project designed to help improve our understanding of post-fire community resilience. Central to the project will be the development of an RFID sensor network which will help support post-fire assessments of water infrastructure damage. These sensors will be deployed across a community’s freshwater pipeline network and will transmit data (e.g., temperature reached, pipeline material, exposure duration) that will help determine whether or not toxins have started leaching from the pipes into the freshwater supply. The current phase of the project focuses on Santa Rosa and Paradise in California where the 2017 Tubbs Fire and the 2018 Camp Fire caused damage to the water distribution systems, resulting in contaminated water within the system.
To help support this effort, CBI has developed a web application called the Wildfire Vulnerability Explorer, which can be accessed at https://wildfirevulnerability.eemsonline.org. This application allows users to explore a set of spatially explicit models developed for Santa Rosa and Paradise that identify areas likely to be vulnerable to water contamination exposure following a large-scale fire event. Estimates of vulnerability are based on three primary factors: the probability of water contamination, socioeconomic sensitivity, and adaptive capacity. The Wildfire Vulnerability Explorer brings this information together in an interactive map in order to help officials with both pre-fire planning and post-fire prioritization of recovery efforts – by identifying communities that are the most vulnerable to water contamination exposure, efforts can be taken to better plan for and direct resources to those areas.
Additional information about the project is available on the OSU project page at the link below:
Sensor Technology for Improved Wildland Urban Interface (WUI) Fire Resilience
Funding for the project was provided by the Alfred P. Sloan Foundation.
Photo courtesy of NASA (https://earthobservatory.nasa.gov/images/144225/camp-fire-rages-in-california): Camp Fire in Paradise, California, which is one of the project study areas.
Conservation Biology Institute is a partner in a new $1 million grant from a new interdisciplinary NSF program to foster building an “open knowledge network.” The inspiration for this type of network comes from Tim Berners-Lee’s (best known founder of the World-wide Web) vision for the “semantic web,” which applies tags with relationships to information on the Internet, allowing computers to do basic reasoning for improving search results and answering questions. Apple’s Siri, Amazon’s Alexa, and Google’s Assistant all use these technologies.
Dr. John Gallo co-wrote the proposal and leads CBI’s participation in the team of 13 researchers and practitioners from 10 other institutions. The team is focused on improving access and contributions to tools for analyzing geographic data called spatial decision support systems. “The proliferation of online mapping technologies has greatly increased access to and utility of these kinds of tools, and a logical next step is increasing our ability to find the appropriate data and tools for your problem and link these together for more complex analyses,” says Principal Investigator Sean Gordon of Portland State University. Through engaging stakeholders in three applied case studies (the management of wildland fire, water quality, and biodiversity conservation), the interdisciplinary project team will develop and test participatory and automated methods for finding and sharing decision-relevant information using semantic web technologies.
The new NSF Convergence Accelerator program is named for its focus on bringing together interdisciplinary teams to address one of NSF’s 10 big ideas, specifically “Harnessing the Data Revolution“, also known as building an Open Knowledge Network. Eighteen other of these phase 1 grants were made, covering areas from molecular manufacturing to tracking potentially disruptive solar phenomena. The “accelerator” part comes from the short time frame. “The application required a 3-week turn around, which is very quick for a NSF grant,” Gordon said. “Our success was largely due to having formed the Spatial Decision Support Consortium, a professional networking group four years ago, so we had ideas and people ready to go.” Each phase 1 project is eligible to submit a phase 2 proposal for up to $5 million by next March, and the process will include giving a short “pitch” talk to a panel of experts and potential funders, much like a venture capital approach.
*Learn more about this ongoing project here.
The USDA Conservation Reserve Program (CRP) works with farmers and landowners to implement conservation management practices on enrolled lands, with paid contracts ranging from 10 to 15 years in length. The CRP Grasslands practices target restoration of agricultural grassland systems by augmenting native vegetation for pollinators, providing habitat for grassland plants and animals, increasing biodiversity, reducing soil erosion, and improving water quality. The USDA’s CRP has been successful in improving the conservation value of millions of acres of farmlands; however, the program currently lacks spatially explicit information on land cover and vegetation within CRP-enrolled tracts.
In partnership with the USDA FSA program, the Conservation Biology Institute (CBI) used a combination of remote sensing and machine learning algorithms deployed on the innovative cloud-computing platform, Google Earth Engine, to map grassland characteristics. We used a rich suite of enviro-climatic data, multiple sources of satellite imagery, and Random Forest modeling techniques to predict land cover for study areas in Washington, Colorado, and Kansas, where CRP Grasslands holdings are most prevalent. We used machine learning to create predictive maps of vegetation type by leveraging an extensive set of satellite-derived variables, environmental layers, and federal survey data (from BLM’s AIM and USDA NRCS’s NRI programs). Our initial investigation utilized Landsat 8 satellite data to model vegetation cover across the Washington study area and then scaled up to the Colorado-Kansas study area. The Washington study site was selected for further model enhancements and an in-depth comparison of Landsat 8, Sentinel-2, and MODIS satellite imagery, to evaluate differences in model development and performance among sensor types. We generated vegetation cover predictions for the year 2019 using Random Forest classification models. Classified outputs for the five vegetation cover models – annual grass, perennial grass, annual forb, perennial forb, and bare soil – were post-processed to exclude water and urban land cover and areas that were not relevant for mapping grasslands.
Mapped outputs showing vegetation percent cover predictions from our pilot project have been integrated into CBI’s CRP online decision support tool. This online tool offers functionality for managers and landowners to view, filter, compare and summarize geospatial information relevant for assessing CRP tracts in the study areas. You will need permission from USDA to use the tool, but it is available at https://crptool.org/. Anyone can view the design of the tool at USDA CRPtool.
You can read more details in the following publication.
Degagne, Rebecca; Pizzino, Declan; Friedrich, Hannah; Gough, Mike; Joseph, Gladwin; Strittholt, James; et al. (2022): Mapping Conservation Reserve Program Grasslands in Washington, Colorado, and Kansas with Remote Sensing and Machine Learning. figshare. Journal contribution. https://doi.org/10.6084/m9.figshare.19141853.v1
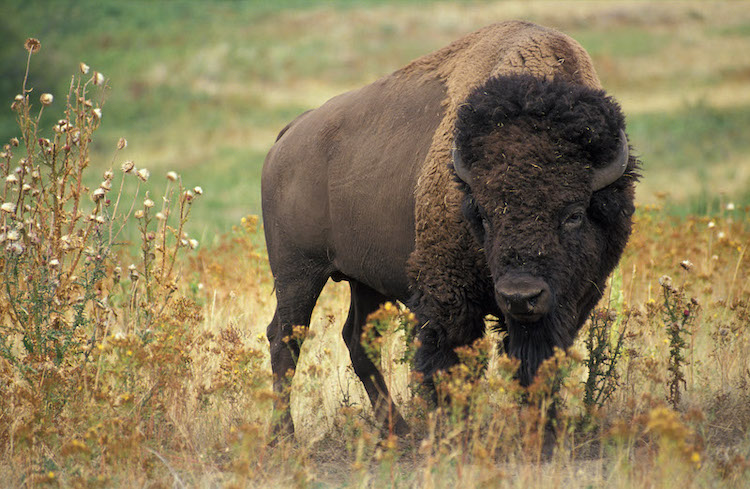
Cachuma Resource Conservation District (RCD) is working in partnership with Conservation Biology Institute, LegacyWorks, and Sharyn Main Consulting on the Regional Priority Plan to Reduce Wildfire Risk and Improve Forest Health in Santa Barbara County (RPP), which is a flagship project of the Santa Barbara County Conservation Blueprint. Funded by the California Coastal Conservancy, the RPP helps with the planning, mapping, and prioritization of projects that will proactively address wildfire threat in Santa Barbara County, as well as improve forest and habitat health.
The RPP is a multi-prong collaboration, which focuses on public and private land in the wildland/urban interface (WUI). CBI is the lead for a component of the project, to develop a sophisticated decision-support mapping tool to not only predict areas of high fire-risk on a landscape scale, but also help the community prioritize where fire risk mitigation projects should occur. The tool will serve as a community resource within the Santa Barbara County Conservation Blueprint and should improve communication, network building, and support community priorities through a regional approach to fire resilience and habitat health. The project team is talking with the many agencies and stakeholders involved, and facilitating the collaborative decision-making process.
Primary forests make up approximately one-third of the world’s remaining forests. Globally, they are grossly under-represented in protected areas and subject to industrial-scale logging and “sustainable forest management” that otherwise fragment intact areas. They face unprecedented threats from logging, mining, energy development, and climate change. British Columbia contains two globally important temperate rainforests with substantial primary and intact forest landscapes distributed from the coast (i.e. Great Bear Rainforest – GBR) inland (i.e., Inland Rainforest). Geos Institute proposes to map and assess conservation importance of the primary forests in these regions as a scientific basis for an international campaign aimed at protecting these globally important rainforests. CBI will support Geos Institute and partners in Canada to help map the area accurately, provide scientific input, and also do a carbon flux model for these primary rainforests.
Wildfires are a natural part of California ecosystems and play an important role in maintaining ecological structure and function. However, different fire regimes in the state have been altered due to past management practices, climate change, invasive species, and population growth and urban expansion. Given the potential for conflicts between fire management and conserving biodiversity and ecological functioning, solutions are needed to balance ecosystem health with human welfare and community safety.
CBI is partnering with Dr. Jon Keeley (USGS) and an international team of landscape ecologists, biologists, geographers and economists to study the factors that control wildfire activity in southern California, which experiences the largest number of housing losses to wildfire in the U.S. This work focuses on the role of different ignition sources, climate patterns, vegetation change, and housing location and arrangement in altering fire patterns and contributing to housing loss at the wildland-urban interface. Other topics of research include the effectiveness and effects of fuel treatments and prescribed fire in controlling large fire behavior; the role of homeowner practices, such as minimizing vegetation around homes and upgrading building construction materials to prevent house loss; and the relative importance of land planning decisions that could best minimize housing loss while preventing negative impacts to biodiversity.
While the research takes place primarily in southern California, the findings are applicable to other fire-prone non-forested ecosystems such as the Great Basin and the other Mediterranean-climate ecosystems across the world.
The results of this research are shared with management agencies like the National Park Service and U.S. Forest Service, in addition to local and state planners and policy makers, to identify the best strategies to increase community safety while minimizing effects on natural ecosystems.
nflicts between fire management and biodiversity conservation
Destructive wildfires are sudden, extreme events: In a matter of hours, both social and ecological communities are transformed by the loss of homes and lives, and change in natural vegetation. After such an event, residents take stock of their transformed landscape and environment, deciding to remain, rebuild, or move, while ecological communities restructure and regrow. These combined social and ecological responses to wildfire may present a ‘hot moment’ or ‘window of opportunity’ where governments, communities, and residents can take action to reduce the future exposure to disaster.
An interdisciplinary team of researchers, convened by CBI’s Dr. Alexandra Syphard, Dr. Miranda Mockrin from Northern Research Station, USDA Forest Service and Dr. Van Butsic, from the Department of Environmental Science, Policy, & Management at University of California, Berkeley, are examining the question “Do wildfires lead to transformative adaptation, reducing future wildfire risk or do they lead to entrenchment, as residents and institutions re-create hazard-prone environments?”
To examine this question, they will review national data of post-fire housing change (rebuilding, sales, new development, land subdivision) and investigate how social and ecological settings and impacts, as well as event characteristics, influence subsequent housing and ecological trends. They will also determine, at the household scale, how changes in housing patterns relate to the post-wildfire ecological setting and socioeconomic characteristics, determining adaptation or entrenchment.
Wildfire is an important ecological process in California, where a diversity of fire regimes shape the structure and composition of plant and animal communities. Fire regimes are changing beyond their historical range of variability, however, due to several factors, including past fire management, invasive species, land use change, and climate change. These changes not only threaten the integrity and diversity of biological communities, but affect human communities, too, as residential losses to wildfire have skyrocketed in the last several decades. Two of these drivers, land use and climate, are expected to change dramatically in the coming century, raising substantial concern about their effects on fire regimes and subsequent impacts to human communities and biological diversity.
CBI has partnered with University of California, Berkeley to develop and implement a scenario-based integrated modeling framework to quantify the relative importance of climatic and land use factors on current and future projected fire patterns and risk of structure loss for three study areas in California. Select research questions driving this project include:
1) How do patterns of fire activity vary by land use change and climate?
2) How does structure loss vary by land use and climate change?
3) How do these relationships varyby geographic region?
4) Given these relationships, how are large fires and associated structure risk likely to change in the future?
Results of the assembled model output will be distributed to appropriate stakeholders and Data Basin will host the mapped output data.
CBI is collaborating with the United States Department of Agriculture (USDA) and the Farm Service Agency to produce an online application depicting ecological and economic features across Bottomland Hardwood Forest Conservation Reserve Program lands in the state of Mississippi. The Conservation Reserve Program (CRP) pays landowners to maintain these Bottomland Hardwood Forests providing important ecological benefits such as removing nitrogen and phosphorus from water, storing flood waters and reducing downstream flooding, trapping sediment, and promoting carbon sequestration. These benefits provided by the CRP are in addition to the restoration and enhancement of wetlands and wildlife habitat. The key ecological and economic features across these CRP lands will be estimated using remote sensing satellite imagery from the Sentinel satellite platform and machine learning modeling using a random forest approach. Additionally CBI staff will be conducting on the ground assessments of the ecological metrics during the 2019 field season.
By providing an online platform that provides metrics on these CRP lands, the USDA Farm Service Agency will be able to better monitor and evaluate existing acres of Bottomland Hardwood Forests that are part of the Conservation Reserve Program. This project is a pilot to determine the utility of the online platform and remote sensing methods, which if proven useful can be expanded to all regions where the CRP restores and enhances Bottomland Hardwood Forests.
The Random Forest modelling process was used to estimate various forest biometric measurements like biomass, density, height, etc., for CRP lands in Mississippi. These were also converted to economic values using standard procedures. We used Forest Inventory and Assessment (FIA) as training data and used field samples to augment the validation of the modelling process. Predicated outputs collected All these outputs were spatialized and incorporated into a customized tool for the USDA Conservation Reserve program.
The CRP tool allows USDA staff, land owners, and third-party organizations to view pertinent spatial information and guide decision making in relation to the status of CRP farms in Mississippi state. This tool allows one to summarize, filter and compare CRP farm tracts across counties and watersheds. You can also download reports in either of two appropriate formats (PDF or CSV). We have also included three different base layers, and relevant contextual data layers that you can view in relation to the CRP farm tracts. You will need permission from USDA to use the tool, but it is available at www.crptool.org. Anyone can view the design of the tool at https://www.sketch.com/s/feba6e2a-ff3e-4c3c-8d2d-9ea4f6bdc896.
CBI initially developed predictive maps of tree height, tree density, biomass, basal area, and forest type using Random Forest machine learning models. Numerous satellite-derived indices from the European Space Agency’s (ESA) Sentinel-1 and Sentinel-2 sensors, in addition to soils and topography data, were used as predictor inputs. We then refined these predictive models, focusing primarily on biomass improvements, by implementing new methods for processing Sentinel-1 imagery on the cloud computing platform Google Earth Engine (GEE); significantly updating model code; and incorporating preliminary data products derived from NASA’s spaceborne LiDAR mission – the Global Ecosystem Dynamics Investigation (GEDI). We refined the GEDI LiDAR-derived data products and included them in our models, and overall accuracy for the four forest regression models ranged from 57% to 91%. The Biomass model saw the greatest improvement in accuracy with the R2 increasing by 8%, from 49% to 57%. The Basal Area and Tree Height models both had minor 1-2% increases in accuracy, while the Tree Density model had no improvement. The Forest Type classification model had a negligible improvement in overall accuracy, however, the Elm/Ash/Cottonwood class increased in accuracy by ~6%, from 64% to 70%.
You can get more details in this publication. Degagne, R., Pizzino, D., Friedrich, H, Gough, M., Joseph, G., Iovanna, R., Smith, C. and Strittholt, J. 2022. Mississippi CRP Forest Remote Sensing with Preliminary Global Ecosystem and Dynamics (GEDI) Mission Derived Data Products. CBI Technical Report 2022-1. 40 pp. (10.6084/m9.figshare.19142147)