Remote Sensing, Cloud Computing and Machine Learning to Characterize Conservation Reserve Program Lands in Washington, Colorado, Kansas and Mississippi
The USDA Conservation Reserve Program (CRP) works with farmers and landowners to implement conservation management practices on enrolled lands, with paid contracts ranging from 10 to 15 years in length. The CRP Grasslands practices target restoration of agricultural grassland systems by augmenting native vegetation for pollinators, providing habitat for grassland plants and animals, increasing biodiversity, reducing soil erosion, and improving water quality. The USDA’s CRP has been successful in improving the conservation value of millions of acres of farmlands; however, the program currently lacks spatially explicit information on land cover and vegetation within CRP-enrolled tracts.
In partnership with the USDA FSA program, the Conservation Biology Institute (CBI) used a combination of remote sensing and machine learning algorithms deployed on the innovative cloud-computing platform, Google Earth Engine, to map grassland characteristics. We used a rich suite of enviro-climatic data, multiple sources of satellite imagery, and Random Forest modeling techniques to predict land cover for study areas in Washington, Colorado, and Kansas, where CRP Grasslands holdings are most prevalent. We used machine learning to create predictive maps of vegetation type by leveraging an extensive set of satellite-derived variables, environmental layers, and federal survey data (from BLM’s AIM and USDA NRCS’s NRI programs). Our initial investigation utilized Landsat 8 satellite data to model vegetation cover across the Washington study area and then scaled up to the Colorado-Kansas study area. The Washington study site was selected for further model enhancements and an in-depth comparison of Landsat 8, Sentinel-2, and MODIS satellite imagery, to evaluate differences in model development and performance among sensor types. We generated vegetation cover predictions for the year 2019 using Random Forest classification models. Classified outputs for the five vegetation cover models – annual grass, perennial grass, annual forb, perennial forb, and bare soil – were post-processed to exclude water and urban land cover and areas that were not relevant for mapping grasslands.
Mapped outputs showing vegetation percent cover predictions from our pilot project have been integrated into CBI’s CRP online decision support tool. This online tool offers functionality for managers and landowners to view, filter, compare and summarize geospatial information relevant for assessing CRP tracts in the study areas. You will need permission from USDA to use the tool, but it is available at https://crptool.org/. Anyone can view the design of the tool at USDA CRPtool.
You can read more details in the following publication.
Degagne, Rebecca; Pizzino, Declan; Friedrich, Hannah; Gough, Mike; Joseph, Gladwin; Strittholt, James; et al. (2022): Mapping Conservation Reserve Program Grasslands in Washington, Colorado, and Kansas with Remote Sensing and Machine Learning. figshare. Journal contribution. https://doi.org/10.6084/m9.figshare.19141853.v1
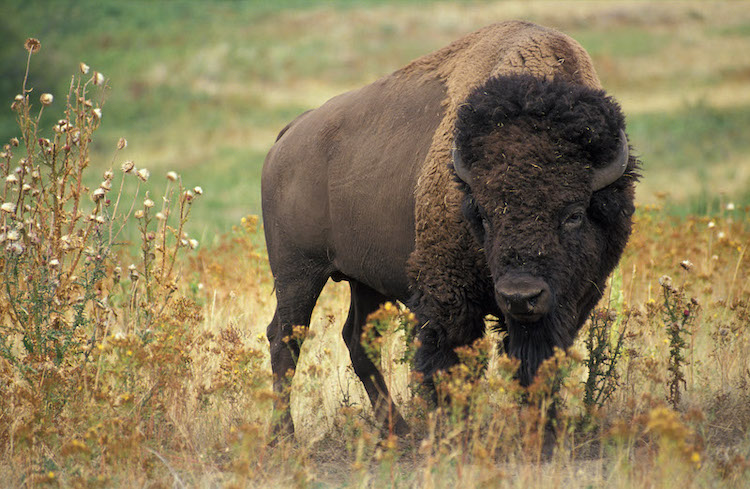